Auto
Tesla Self-Driving System Analyst Nearly Crashes. Is The Dream Over?
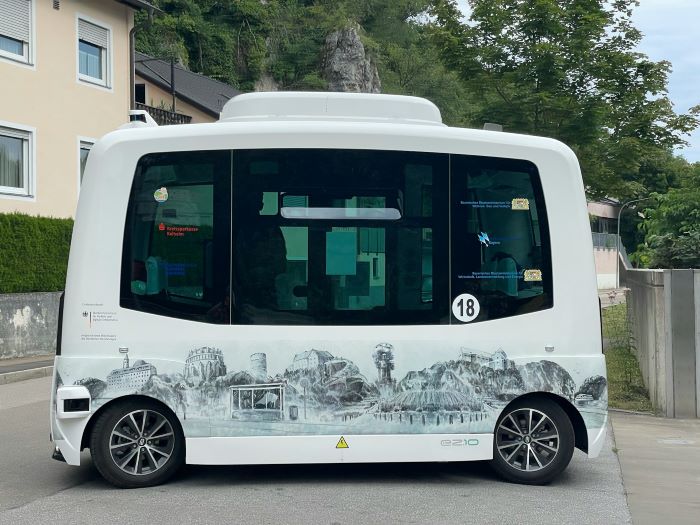
Tesla began developing a self-driving system for its electric vehicles ten years ago. Back then, expectations were high and many industry insiders, including executives at the company, believed that full autonomy was within reach.
Fast forward a decade, and that’s patently not the case (despite the number of patents filed by the Texas-based automaker). Tesla vehicles still can’t navigate the roads like humans because machines can’t learn all the “corner cases” or random mishaps that take place on the road.
This issue came to a head in a recent story where a Tesla analyst nearly crashed in a Model S with full self-driving activated. The driver was driving on a sunny, normal day with his son in the car. The idea was to test the car’s full-self-driving (FSD) capabilities to see whether the automaker was any closer to autonomy.
Unfortunately, the test didn’t go as planned. During the FSD analysis, the vehicle accelerated into an intersection and failed to follow police instructions, ignoring the officer standing in the road. The tester revealed that this situation was dangerous and sent a letter to Tesla to describe his findings and how the vehicle required improvement. The company sent a short response but didn’t clarify whether they were actively working on the issues raised.
Auto Claims Assist, a company that helps people with the car accident claims process says that machine technology still poses a risk. “Software developments are gathering pace, but the ability of learning algorithms to react to untrained data remains limited. The media believes that AI systems possess human-like intelligence, but that’s quite far from the truth. If they did, more self-driving cars would be able to understand context and react to novel challenges on the road.”
Granted, test analyst William Stein was using a slightly older version of the company’s software. But the key point remains that corner solutions remain an issue for Tesla. The company (like other AI developers) can’t overcome the issue.
Even six years ago in 2018, the major problem was unexpected roadside events that weren’t present in training data. Elon Musk talked about how his team was trying to accommodate as many of these as possible but the number of possible combinations of events are tremendous and no AI system can accommodate them all.
For this reason, Tesla invests heavily in putting cameras inside its vehicles to watch drivers to ensure they pay attention to the road ahead. Systems watch to see if drivers are focusing on the road and not looking around down by their sides. It also insists they keep their hands near the wheel, just in case they need to take over.
“We see a lot of people in our business who want protection from motor vehicle accidents,” Auto Claims Assist says. “It will be interesting to see whether machine-driven vehicles eventually operate under different rules and who will ultimately take responsibility. At the moment, it seems like the driver is still in control, but that may change in the future.”
Why Full Self-Driving Vehicles Is So Challenging
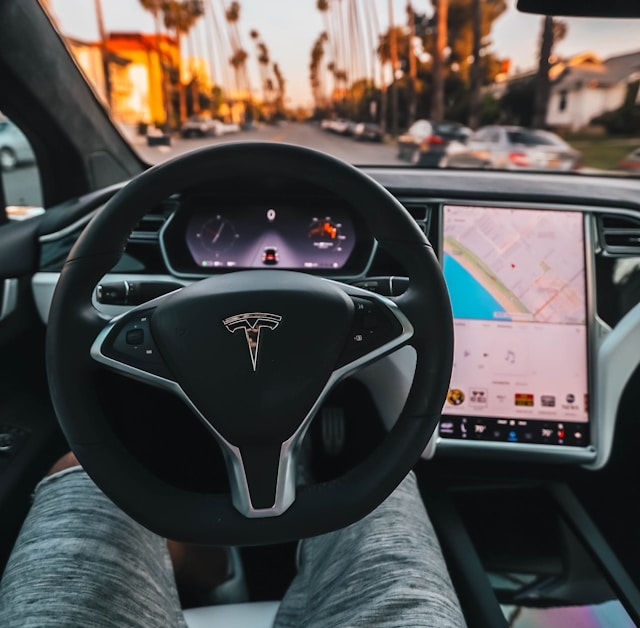
Source: Unsplash
This all begs the question, why are full self-driving vehicles so challenging to manufacture? What is it about driving that’s so difficult for algorithms and software to replicate?
Complexity
The top issue is complexity. Machines have to parse an almost infinite number of situations, some of which they may never see in their training data.
Currently, self-driving systems work 99% of the time. But that’s not good enough for the road. Regulators want to see that systems always make the correct choice, regardless of circumstances or how they compare to human drivers.
For this reason, companies like Tesla and Google are having to manually program certain situations into their machines to help them navigate them in the future. Many are focusing on edge cases, trying to eliminate situations that might cause vehicles to make a mistake.
But, unfortunately, there are an infinite number of these, and the software can’t always decide what to do next. One issue is animals entering the road. Another is the idea that children might follow a ball rolling into the street. Humans understand this context and can make linkages, but machines trained on visual data alone without an underlying structure of how the world works can’t do this.
Sensor Limitations
On a more superficial level, there are also serious sensor limitations on many of these vehicles. Car companies are having to use a combination of radar, LIDAR, and cameras to understand the environment and make better decisions. All this incoming data requires high-powered computers to process everything to come to statistical likelihoods about what might happen next.
Sensors, for instance, don’t work well in rain and fog. Night driving is also an issue for some systems (particularly normal-light cameras). Computers can also mistake inputs, changing how the car behaves.
Challenges Getting Algorithms To Reflect The Real World
There are also serious and deep issues related to getting algorithms to match the real world. While some might be able to approximate it, it is hard for them to capture it closely enough for it to be practical.
One problem is the size of many of the deep learning models being trained for use in vehicles. Many systems are enormous and require massive datasets that make it prohibitively expensive to compute them. Accuracy or safety might also be a factor, especially if these systems are being used in real time. Cars might not have sufficient time to calculate optimal routes.
Public Trust
Finally, public trust is an issue. Many people aren’t ready to give up on human drivers yet and rely solely on AI. The technology remains unproven, but it is challenging to see how it will be proved if car companies, like Tesla, continue to struggle with safety.
Conclusion
In conclusion, Tesla’s self-driving vehicles might still be some distance from full autonomy. Self-driving still needs a quantum leap in computer reasoning and understanding of the world, beyond what’s already been done. Systems should have a kind of common sense that allows them to react appropriately to all situations, not just those in their training set.