Finance
Mastering the Market: Tips for Implementing Effective Oil Trading Algorithms
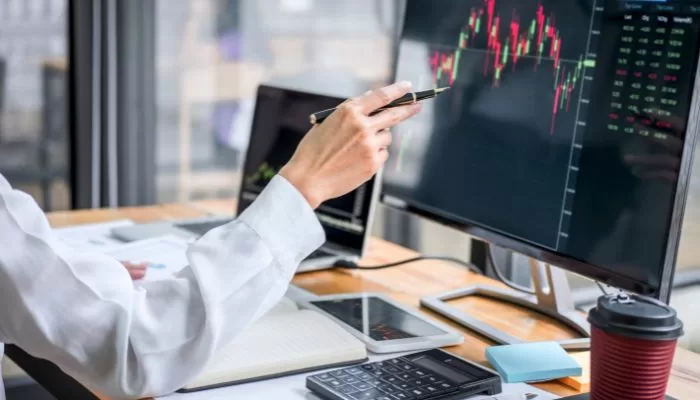
Oil trading algorithms are sophisticated sets of rules designed to guide trading decisions in the oil market. These algorithms leverage historical data, market trends, and other relevant factors to make informed trading decisions. In the modern trading landscape, algorithms play a crucial role in executing trades efficiently and profitably. Oil trading can be tedious and especially for beginners! Go oil-profits.com to understand the right approach for oil investing and that too from the experts.
Key Components of Successful Oil Trading Algorithms
Successful oil trading algorithms rely on several key components. First, they require access to reliable data sources, including real-time market data, historical price data, and fundamental data such as supply and demand metrics. Algorithms also need robust analysis methods to interpret this data accurately and make informed trading decisions.
Another critical component is the design and implementation of the algorithm itself. This involves creating a set of rules that define how the algorithm will operate, including when to enter and exit trades, how to manage risk, and how to adapt to changing market conditions. The algorithm must also be tested rigorously to ensure its effectiveness in different market scenarios.
Utilizing Machine Learning and AI for Enhanced Algorithmic Trading
Machine learning and artificial intelligence (AI) have revolutionized algorithmic trading in the oil market. These technologies enable algorithms to learn from past data and improve their performance over time. Machine learning algorithms can analyze large datasets to identify patterns and trends that human traders may overlook, leading to more profitable trading strategies.
AI-driven algorithms can also adapt to changing market conditions in real-time, allowing them to make quick and accurate trading decisions. This capability is especially valuable in the volatile oil market, where prices can fluctuate rapidly based on geopolitical events, economic indicators, and other factors.
Risk Management Strategies for Algorithmic Trading
Risk management is a critical aspect of algorithmic trading in the oil market. Effective risk management strategies help mitigate potential losses and protect trading capital. One common risk management technique is to set stop-loss orders, which automatically close a trade if the price moves against the trader beyond a certain threshold.
Another important risk management strategy is diversification, which involves spreading trading capital across different assets or markets to reduce the impact of any single trade or market event. Additionally, algorithms can incorporate risk-reward ratios into their trading decisions, ensuring that potential profits outweigh potential losses.
Optimizing Performance Through Backtesting and Simulation
Backtesting and simulation are essential tools for optimizing the performance of oil trading algorithms. Backtesting involves testing an algorithm against historical data to evaluate its performance under various market conditions. This helps traders identify potential flaws in the algorithm and make improvements before deploying it in live trading.
Simulation, on the other hand, involves running the algorithm in a simulated trading environment that mimics real market conditions. This allows traders to see how the algorithm would perform in real-time without risking actual capital. By fine-tuning their algorithms through backtesting and simulation, traders can improve their chances of success in the oil market.
Compliance and Regulatory Considerations
Algorithmic trading in the oil market is subject to regulatory oversight to ensure fair and orderly trading. Traders must adhere to regulations set forth by regulatory bodies such as the Commodity Futures Trading Commission (CFTC) in the United States. These regulations often include requirements for algorithmic trading, such as the need for pre-trade risk controls and monitoring systems.
Traders must also consider ethical considerations when developing and implementing algorithms. They should ensure that their algorithms are not designed to manipulate markets or engage in unfair trading practices.
Case Studies: Successful Implementations of Oil Trading Algorithms
Several successful implementations of oil trading algorithms demonstrate their effectiveness in the market. For example, a hedge fund used a machine learning algorithm to analyze satellite images of oil storage tanks to predict changes in oil inventories accurately. This allowed the fund to make timely trading decisions based on real-time data.
In another case, a trading firm used an algorithmic trading strategy based on statistical arbitrage to profit from price inefficiencies in the oil market. By leveraging historical price data and advanced statistical models, the firm was able to identify profitable trading opportunities and execute trades with minimal risk.
Future Trends and Innovations in Oil Trading Algorithms
The future of oil trading algorithms is likely to be shaped by emerging technologies such as blockchain, big data analytics, and the Internet of Things (IoT). These technologies can provide traders with access to more accurate and timely data, allowing them to make better-informed trading decisions.
Additionally, advancements in AI and machine learning are expected to further enhance the capabilities of oil trading algorithms. These technologies will enable algorithms to analyze larger datasets, identify more complex patterns, and make more accurate predictions, leading to increased profitability for traders.
Conclusion
In conclusion, mastering the market through effective oil trading algorithms requires a deep understanding of the market, the use of advanced technologies, and rigorous risk management strategies. By incorporating these tips into their trading strategies, traders can improve their chances of success in the dynamic and challenging oil market.